Digital Assessment in the Big Data Era
- jessikaledezma
- Aug 11, 2024
- 8 min read

Image retrieved from Canva
The Impact of Big Data on Education
As I have mentioned in my previous blog, technology is changing how we learn and, as educators, how we present new concepts to students. The rise of AI and new technologies has completely changed how we gather and use data in education. Not too long ago, collecting data took much work. Just think of paper tests and handwritten grade books. It was time-consuming and often limited in what it could tell us about student performance. Now, because of AI and other programs, educators can collect and analyze vast amounts of data quickly and accurately. This technology lets them see patterns, track progress, and make decisions that are much more informed and effective. Big data rapidly transforms education, leading to a new era where technology-driven insights shape how students are assessed, graded, and supported throughout their learning journey. As discussed in the article “Big Data in Education," posted by Maryville University, "Big data is transforming how schools analyze information and make decisions in areas such as academic performance, faculty effectiveness, organizational outreach, and technology efficiency" (2020). Analyzing large amounts of data allows educators to improve their teaching methods, provide personalized learning experiences, and maintain academic integrity more effectively.
Machine-Graded Assessments
The introduction of machine-graded assessments marks a significant evolution in the educational landscape. Using sophisticated algorithms can help these systems quickly and accurately evaluate student responses to various questions. This technology offers considerable benefits, particularly in terms of efficiency and consistency. Educators can now process large volumes of student work with minimal delay, allowing them to focus more on instruction and less on the labor-intensive task of grading. Moreover, machine grading minimizes the risk of human error and bias, ensuring that all students are evaluated somewhat based on their performance. However, while machine grading excels in handling objective questions such as multiple-choice or true/false items, it faces challenges regarding subjective, open-ended responses. As stated by Xie (2024), "While large language models (LLMs) have been used for automated grading, they have not yet achieved the same level of performance as humans, especially when it comes to grading complex questions.” Essays, projects, and creative assignments may require detailed evaluation that machines may not yet fully replicate, highlighting the need for a balanced approach that combines the strengths of both human and machine grading, at least for now.
Computer-Based Text Analysis (CBTA)
The future of big data in education is connected with technological advancements. With the right tools, educators can assess students in diverse ways that address varying skill levels within the classroom. The field of computer-based testing encompasses a multitude of acronyms, presenting a potentially overwhelming landscape. Aken (2017) states, "Most CBTA programs are developed to assess written text to provide a summary score (summative assessment). Some applications, however, are primarily as a learning environment designed to assist students in learning how to write (formative assessment) as well as possibly providing summative assessment.” Akens (2017) explores Computer-Based Text Analysis (CBTA) techniques, which automate text evaluation. These techniques are divided into two main categories: Text in Isolation (TIl) and Corpus-Based Analysis (CBA). TIl methods assess text independently, while CBA methods compare the text to a pre-existing corpus for contextual understanding. Each category includes various nuanced approaches, offering diverse methods for evaluating student writing. CBTA techniques enhance the accuracy and efficiency of text analysis in educational settings. As technology evolves, we expect more sophisticated applications integrating data analytics, artificial intelligence, and machine learning to enhance educational assessments. These advancements will allow for more granular insights into student performance and learning patterns, facilitating the development of highly personalized learning experiences. This data-driven approach not only supports educators in refining their instructional strategies but also contributes to a more adaptive and inclusive educational system, where teaching methods and assessment tools are continually evolving to meet the needs of all learners.
(Aken, 2017)
Computer Adaptive Testing (CAT)
Computer Adaptive Testing (CAT) tailors questions to each student's knowledge level. As explained by Cope and Kalantzis (2016), "Computer adaptive tests serve students progressively harder or easier questions depending on whether they answer correctly." This approach provides educators with more meaningful feedback and reduces the likelihood of cheating, as no two students will likely encounter the same questions. By dynamically adjusting the difficulty of questions based on a student's responses, CAT ensures that each learner is challenged appropriately, fostering deeper engagement and reducing frustration. As discussed by Cope and Kalantzis (2016), “These testing processes and technologies are increasingly embedded into pedagogical practice, for instance, in the form of end-of-chapter tests in e-textbooks, comprehension tests in online reading programs, or quizzes delivered through learning management systems” (p.3). Moreover, this individualized approach helps identify specific areas where a student may need additional support, allowing educators to tailor their instruction more effectively. Additionally, CAT's adaptability can lead to more accurate assessments of a student's true abilities, as it minimizes the impact of guessing or test anxiety, which can often skew results in traditional testing formats. As a result, CAT supports a more equitable and responsive educational environment, where assessments are not just a measure of what students know but also a tool for guiding their ongoing learning journey.
Natural Language Processing (NLP)
As stated by Jim Holdsworth (2024), "Natural language processing (NLP) is a subfield of computer science and artificial intelligence (AI) that uses machine learning to enable computers to understand and communicate with human language. NLP enables computers and digital devices to recognize, understand, and generate text and speech by combining computational linguistics—the rule-based modeling of human language—with statistical modeling, machine learning (ML), and deep learning.” In the context of assessments, many CAT systems use NLP to evaluate written responses through two primary methods: statistical corpus comparison and analytical text parsing (Cope & Kalantzis, 2016). In statistical corpus comparison, the system is trained by comparing human-graded texts with student responses to determine a grade. On the other hand, analytical text parsing involves programming the machine to identify specific features in the writing that demonstrate subject competency.
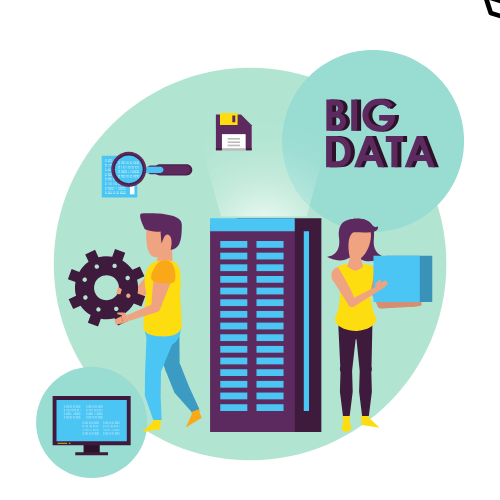
Image retrieved from Canva
Online Grading
The shift to online grading systems has revolutionized how assessments are managed and communicated. As stated by O'Donnell (2018) “No, not just all those fill-in-the bubble multiple choice questions. The longer answers and essays too." Now, digital platforms allow educators to grade assignments, exams, and quizzes electronically, often with the added benefit of integrating effortlessly with learning management systems (LMS). This integration streamlines the grading process, from submission to feedback, making it faster and more efficient. Artificial intelligence (AI) advances have significantly impacted grading, with AI now handling the grading. For instance, as discussed by O'Donnell (2018), in Ohio, "computers are now scoring the entire test for about 75 percent of students," as noted by State Superintendent Paolo De Maria and state testing official Brian Roget. "The other 25 percent are scored by people to help verify the computer's work." This balance between automated and human grading can enhance accuracy while maintaining a human touch in the evaluation process. However, as some have expressed, "I do not know if it is good or not” (O'Donnell,2018), highlighting the ongoing debate around the effectiveness and implications of relying heavily on technology in education. One of the critical advantages of online grading is the immediate access it provides students. As soon as their work is graded, students can view their scores and receive detailed feedback, which helps to create a more interactive and responsive learning environment. This immediacy helps students understand their strengths and areas for improvement and encourages continuous learning and development. Many online grading systems may include advanced features such as plagiarism detection tools. These tools play a crucial role in maintaining academic integrity by identifying potential instances of copying or unauthorized collaboration. However, the reliance on digital platforms for grading also raises concerns about data privacy and the security of sensitive student information. Institutions must ensure that these systems are robust and secure, protecting both the integrity of the grading process and the confidentiality of student records.
Academic Honesty
As education increasingly moves online, the challenge of maintaining academic integrity has become more evident. Academic integrity in computer-based assessments refers to the commitment of learners to uphold ethical standards and honesty while engaging with digital testing tools. As Dr. Grandinetti (n.d) stated, "Educators often approach the subject of academic integrity with a negative tone, providing dire warnings of the consequences of misbehavior. However, a positive approach that helps students understand what academic integrity is—and why it matters—is far more empathetic and supportive.” The convenience of digital learning and assessment has brought with it new opportunities for dishonesty, from plagiarism to unauthorized collaboration during exams. In response, educators and institutions are turning to big data-driven tools to uphold academic integrity in the digital age.
As stated by Grandinetti (n.d)
Adopting an integrated approach to academic integrity will help you avoid putting your students in this position. Through thoughtful design, you can foster and maintain a culture of academic integrity that goes beyond simply deterring and detecting cheating.
· First, design your assessments proactively to encourage academic integrity
· Second, deter cheating by communicating clear expectations for academic integrity
· Third, detect academic misconduct with technology tools (if necessary)
Tejada (2023) explains, "As online education continues to gain popularity, ensuring academic integrity in online courses has become a critical concern for educators and institutions.” While computer-based assessments offer various tools to prevent cheating, such as adaptive testing and time limits, true academic integrity ultimately depends on the learner's choices. However, the effectiveness of these measures depends not only on the technology itself but also on the ethical considerations surrounding its use. There is a delicate balance between ensuring academic integrity and respecting student privacy. Excessively rigorous or invasive monitoring can create a climate of mistrust and anxiety, which may negatively impact the learning experience. Therefore, institutions need to implement these tools thoughtfully, alongside efforts to cultivate a culture of integrity and ethical behavior among students. Maintaining academic integrity involves both technological measures and quality instruction, with the latter being particularly crucial in fostering an environment where cheating becomes unnecessary and unappealing to students. Indeed, well-prepared students, through effective teaching, are less likely to resort to dishonest practices.
Conclusion
Big data is reshaping the educational landscape, offering new ways to enhance the assessment process, personalize learning, and uphold academic standards. Implementing machine-graded assessment programs has significantly improved efficiency, accuracy, and accessibility. At the same time, the challenge of maintaining academic integrity in an increasingly digital world has prompted the development of sophisticated tools to detect and deter dishonest behavior. While the benefits of big data in education are substantial, they come with essential considerations that must be carefully managed. Issues related to data security, privacy, and the potential for algorithmic bias require ongoing attention to ensure that the advantages of these technologies are fully realized without compromising ethical standards. As educators and institutions navigate this evolving landscape, the thoughtful integration of big data will be vital to creating a more effective, equitable, and trustworthy educational environment.
Resources for reference:
References
Aken, A. (2017). An evaluation of assessment-oriented computer-based text analysis paradigms. Higher Education Research, 2(4), 111–116. doi: 10.11648/j.her.20170204.12
Big Data in Education. Maryville University Online. (2020, December 7). https://online.maryville.edu/blog/big-data-in-education/
Cope, B., & Kalantzis, M. (2016). Big data comes to school: Implications for learning, assessment, and research. Aera Open, 2(2), 2332858416641907.
Grandinetti, P. (n.d.). Strategies and tools for academic integrity in online environments. Teaching and Learning Resource Center. https://teaching.resources.osu.edu/teaching-topics/strategies-tools-academic-integrity
Holdsworth, J. (2024, June 6). What is NLP (Natural Language Processing)? IBM. https://www.ibm.com/topics/natural-language-processing
O’Donnell, P. (2018, March 19). Computers are now grading essays on Ohio’s state tests. Cleveland. https://www.cleveland.com/metro/2018/03/computers_are_now_grading_essays_on_ohios_state_tests_your_ch.html
Tejada, A. (2023, June 5). Strategies for promoting academic integrity in online courses. eLearning Industry. https://elearningindustry.com/strategies-for-promoting-academic-integrity-in-online-courses
Xie, W. (2024, May 30). Grade Like a Human: Rethinking Automated Assessment with Large Language Models. Grade like a human: Rethinking automated assessment with large language models. https://arxiv.org/html/2405.19694v1#S3
Hello Jessika,
To begin, I appreciate your insights on the reading. You started off crafting a memory of a time that educators can relate to, the time-consuming task of collecting grades. Thankfully, with the advent of AI, that time is better spent focusing on the real needs of the classroom. I feel that the ability to provide personalized learning paths for our students is the greatest advantage afforded since the invention of AI and digital grading. Which of the advantages that you listed do you think saves the most time for teachers? You also mentioned machine-graded assessments and the issues surrounding that. I can only speak for teachers who were in school during the TAAS period and it…
Jessika,
Great job with your blog. Your visuals and videos always add to the aesthetic of your blog , but most importantly add more value to it. Your introduction of the impact of Big Data in education set the stage for your blog and what was to come . You're right educators can collect and analyze vast amounts of data quickly and accurately. I am curious to find out from current educators what their favorite data is to collect and analyze.
Your take on machine grading is well thought out , because you explain that it truly does have great advantages to it, like less work for teachers to have but it also has its challenges. That is important for…
Jessika,
Your blog "Digital Assessment in the Big Data Era" gave a great insight on both topics. I love how you touched on AI technologies. That technology is so new and so rapidly changing! I liked how you wrote about the machine-graded assessments. I do think if done well, they will save time and money. However, I am still skeptical because the technology has not been totally perfected yet. I do believe that essays, projects, and creative assignments still need human touch and eyes when grading. It would be hard for a machine to grade my students taking photos at ballgames and designing yearbook pages.
Your section on CBTA was very well detailed. You do a great job of breaking…